What is Explanatory Variable and Response Variables?
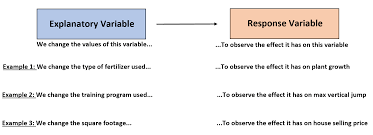
Using experiments or observations, you frequently study causal links between variables in research. For instance, you may investigate whether caffeine enhances speed by administering various doses of caffeine to volunteers and then comparing their reaction times. An explanatory variable is what you alter or observe changes in (e.g., caffeine dose), whereas a response variable is what varies as a result (e.g., blood pressure) (e.g., reaction times). Explanatory variable and response variable are frequently interchangeable with other research terminologies.
Independent variables include explanatory variables. The terms are frequently interchangeable. However, there is a small distinction between the two. When a variable is independent, it has no influence from other variables. When a variable is not absolutely independent, it is referred to as an explanatory variable.
Consider two variables that could explain weight gain: fast food and cola. Although you may believe that fast food consumption and soda use are unrelated, this is not the case. Fast food restaurants encourage you to purchase a Coke with your meal. And if you stop to get a Coke, there are frequently several fast food options, such as nachos and hot dogs. Although these variables are not entirely independent of one another, they do influence weight growth. They are referred to as explanatory variables since they may explain the weight gain.
Typically, the distinction between independent variables and explanatory factors is so inconsequential that nobody cares. Unless you are conducting advanced research involving a large number of factors that can interact. It can be crucial for clinical studies. In the majority of instances, notably in statistics, the two terms are equivalent.
Explanatory vs. response variables
Simple distinction exists between explanatory and response variables:
An explanatory variable is the anticipated cause that explains the observed findings.
A response variable is the anticipated outcome, and it is influenced by explanatory variables.
You anticipate that response variable changes will occur only after explanatory variable modifications.
There exists either an indirect or direct causal relationship between the variables. In an indirect relationship, an explanatory variable may exert influence over a response variable via a mediator.
There are no explanatory and response variables when dealing with a purely correlational relationship. Even though changes in one variable are associated with changes in another variable, both may be the result of a confounding variable.
Variables as examples of explanatory and response
In some studies, there will be simply one explanatory variable and one response variable, but in more complex research, one or more response variables may be predicted using several explanatory factors in a model.
Research question | Explanatory variables | Response variable |
---|---|---|
Does academic motivation predict performance? |
|
|
Can overconfidence and risk perception explain financial risk taking behaviors? |
|
|
Does the weather affect the transmission of Covid-19? |
|
|
Explanatory vs independent factors
There are subtle distinctions between explanatory factors and independent variables despite their similarity.
In the context of research, independent variables are ostensibly unaffected by or reliant on any other variable; they are changed or adjusted alone by researchers. In a controlled experiment where the amount of caffeine each participant takes can be precisely controlled, caffeine dose is an independent variable.
However, the term “explanatory variable” is sometimes preferred to “independent variable” because, in the real world, independent variables are frequently influenced by other variables. This indicates that they are not fully independent.
Example: Explanatory Variables vs. Response Variables
You are examining whether gender and risk perception may explain or predict stress responses to various situations.
You collect a sample of young adults and have them complete a questionnaire in the laboratory. They describe their risk perceptions of various frightening circumstances while you monitor their physiological stress responses.
In your research, you discover a strong correlation between gender and risk perception. Women are more inclined than men to see circumstances as dangerous.
This indicates that gender and risk perception are interdependent. They are more accurately referred to as explanatory variables for the stress reaction response variable.
In regression analyses, which focus on predicting or accounting for changes in response variables as a result of explanatory variables, the words “explanatory variable” and “response variable” are frequently employed.
The response variable is the subject of a study or experiment’s inquiry. A variable that explains the changes in another variable is an explanatory variable. It might be anything that has the potential to influence the response variable.
Consider that you are attempting to determine if chemotherapy or anti-estrogen therapy is the superior treatment for breast cancer patients. The question is: which surgery extends lifespan the most? Therefore, survival time serves as the response variable. The type of treatment administered is the explanatory variable, which may or may not influence the response variable. In this instance, there is only one explanatory variable: treatment type. In real life, there would be more explanatory variables, such as age, health, weight, and other lifestyle characteristics.
A scatterplot can be used to identify trends in paired data. When a response variable and an explanatory variable are present, the explanatory variable is always shown on the x-axis (the horizontal axis). Always plot the response variable on the y-axis (the vertical axis).
If you examine the image above, you should be able to determine that wrist circumference is a poor predictor of body fat (the response variable). The red line in the illustration is the best-fitting line. Although it goes through the centre of the cluster of dots, the majority of the dots are not near it. This indicates that the explanatory variable does not actually explain anything.
However, the size of a person’s thighs is a greater indicator of body fat. Even this is not flawless. Numerous fit individuals have huge thighs! Determine how close the dots are to the best-fitting red line.
Frequently asked questions about explanatory and response variables
What are explanatory and response variables?
Simple distinction exists between explanatory and response variables:
- An explanatory variable is the anticipated cause that explains the observed findings.
- The expected effect of a response variable, which responds to other variables.
How do explanatory variables differ from independent variables?
Because independent variables are sometimes influenced by other variables in real-world contexts, “explanatory variable” is sometimes preferable to “independent variable.” This indicates that they lack complete independence.
Multiple independent variables may also be correlated with one another, therefore the phrase “explanatory variables” is more apt.
How do you plot explanatory and response variables on a graph?
On graphs, the explanatory variable is typically plotted on the x-axis and the response variable on the y-axis.
- Utilise a scatter plot or line graph if you have quantitative variables.
- Utilise a scatter plot or line graph if your answer variable is categorical.
- Utilise a bar graph if your explanatory variable is categorical