Guide to Using ChatGPT For Data Science- Is More Important Than Coding
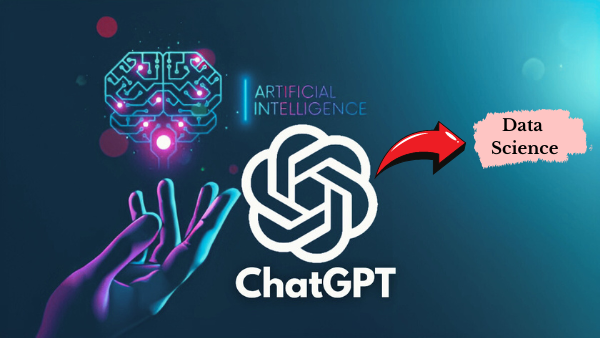
Guide to Using ChatGPT For Data Science: Is It More Important Than Coding? – A Complete Guide
The realm of data science, traditionally dominated by complex algorithms, models, and coding, has seen a transformation with the advent of sophisticated AI models like ChatGPT. The question arises: Is leveraging such models becoming more critical than coding itself? This guide will explore the role of ChatGPT in data science and its comparison with traditional coding. Learn Data Science or improve your skills online today. Choose from a wide range of Data Science Course offered from top universities and industry leaders.
Also Read:- How to Use ChatGPT for Social Media Marketing- 50+ Tips
1. Understanding ChatGPT in the Context of Data Science:
- Natural Language Processing (NLP): ChatGPT’s primary strength lies in its advanced NLP capabilities, making it adept at understanding and generating human-like text.
- Extensive Knowledge Base: With training on vast amounts of data, ChatGPT possesses a wide knowledge base, making it an asset for quick information retrieval or hypothesis generation.
2. How Can Data Scientists Use ChatGPT?
a. Data Exploration:
- Pose questions about datasets, potential correlations, or trends and get insights or summaries.
b. Hypothesis Generation:
- Based on a brief dataset description, ChatGPT can suggest potential hypotheses or questions worth investigating.
c. Algorithm Explanations:
- Get simplified explanations for complex algorithms, aiding in understanding or teaching.
d. Data Cleaning Suggestions:
- Seek advice on preprocessing techniques suitable for specific datasets.
e. Model Recommendations:
- Based on data type and objective, get suggestions for potential modeling techniques.
3. ChatGPT vs. Traditional Coding:
a. Flexibility:
- While ChatGPT can provide quick insights or explanations, coding offers unparalleled flexibility in terms of custom algorithms, data manipulations, and integrations.
b. Depth:
- ChatGPT is great for broad strokes or initial steps in data analysis. In contrast, coding allows for in-depth analysis and fine-tuning.
c. Speed:
- For standard queries or explanations, ChatGPT can be faster. But for detailed data operations, coding is indispensable.
d. Dependency:
- Relying solely on ChatGPT might lead to a dependency that could be limiting in scenarios where bespoke solutions are needed.
4. Balancing ChatGPT with Traditional Data Science Skills:
a. Initial Exploration:
- Use ChatGPT for initial data exploration, understanding algorithms, or brainstorming.
b. Deep Analysis:
- Transition to coding for detailed data transformations, custom visualizations, or model building.
c. Continuous Learning:
- Use ChatGPT as a tool for learning, but continually upskill in traditional data science techniques.
5. The Future: Integration Over Replacement:
- Complementary Use: ChatGPT and traditional coding are best seen as complementary. While ChatGPT can speed up certain aspects, coding remains at the heart of bespoke solutions.
- Automation & Custom Solutions: As AI models advance, we might see more automation in standard tasks, but the need for custom solutions will ensure coding remains integral to data science.
Also Read:- How to Automatically Generate WordPress Posts With ChatGPT
Conclusion
ChatGPT offers exciting possibilities in the world of data science, speeding up processes and making information more accessible. However, it’s crucial to see it as a tool in the data scientist’s arsenal rather than a replacement for coding. The harmonious integration of AI-driven tools like ChatGPT with traditional data science coding skills will pave the way for the future of the domain.