How to Build a Knowledge Graph in 10 Steps
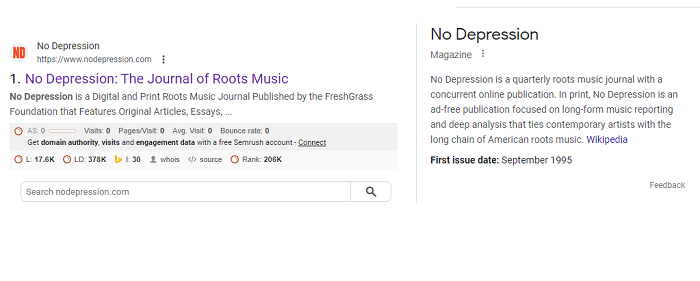
Building a Knowledge Graph (KG) involves creating a structured representation of information, where entities (things, people, places, concepts) are connected by relationships. Google’s Knowledge Graph, for instance, powers its semantic search capabilities. Here’s a comprehensive guide to building your own Knowledge Graph:
Also Read:- How To Create Semantic Content Network? Ranking Websites on Google
1. Define the Scope:
- Purpose: Determine the primary goal of your KG. Is it for an internal project, a specific industry, or a broader audience?
- Entities: Identify the main entities you want to include. For a movie KG, entities could be actors, directors, movies, genres, etc.
2. Data Collection:
- Sources: Identify reliable data sources. This could be databases, websites, APIs, or even manual data entry.
- Data Formats: Data can be in various formats like CSV, JSON, XML, etc. Ensure you have tools to parse these.
3. Data Cleaning:
- Remove Duplicates: Ensure that entities are unique.
- Normalization: Standardize data formats, date representations, etc.
- Validation: Ensure data accuracy and relevance.
4. Entity Recognition:
- NLP Tools: Use Natural Language Processing tools like spaCy or Stanford NER to identify entities from unstructured data.
- Entity Resolution: Different sources might refer to the same entity in different ways. Resolve these to a single, canonical form.
5. Define Relationships:
- Types of Relationships: For a movie KG, relationships could be “acted in,” “directed by,” “belongs to genre,” etc.
- Directionality: Some relationships might be directional. For instance, “A directed B” is not the same as “B directed A.”
6. Knowledge Graph Construction:
- Graph Databases: Tools like Neo4j or OrientDB are popular for building KGs.
- Triple Stores: Represent data in the form of triples (subject, predicate, object), e.g., (Tom Hanks, acted in, Forrest Gump).
7. Integration with External KGs:
- Linking: Integrate with established KGs like DBpedia, Wikidata, or Google’s Knowledge Graph for a richer dataset.
- APIs: Use APIs to fetch and integrate data.
8. User Interface:
- Visualization: Tools like Gephi or Cytoscape can help visualize your KG.
- Search Interface: Implement a search functionality to query the KG.
9. Continuous Updating:
- Monitoring: Regularly check for updates in your data sources.
- Feedback Loop: Allow users to suggest edits or additions to keep the KG current and accurate.
10. Applications:
- Semantic Search: Use the KG to power a search engine that understands context.
- Recommendation Systems: Recommend related entities based on the KG structure.
- Data Analysis: Analyze the KG for insights, patterns, and trends.
11. Ensure Privacy and Compliance:
- Data Permissions: Ensure you have the right to use and display the data.
- Anonymization: If using personal data, ensure it’s anonymized and complies with privacy regulations.
12. Evaluation:
- Accuracy: Regularly evaluate the accuracy of the KG.
- Completeness: Ensure that the KG is comprehensive and covers all entities and relationships within its scope.
Also Read:- How to Use ChatGPT Code Interpreter Feature- Blogs Year
Building a Knowledge Graph is a dynamic process that requires continuous refinement and updating. As more data becomes available and as the domain of knowledge evolves, the KG should evolve with it.